Why Data Quality is the Lifeblood of AI: The Key to Training and Output Excellence
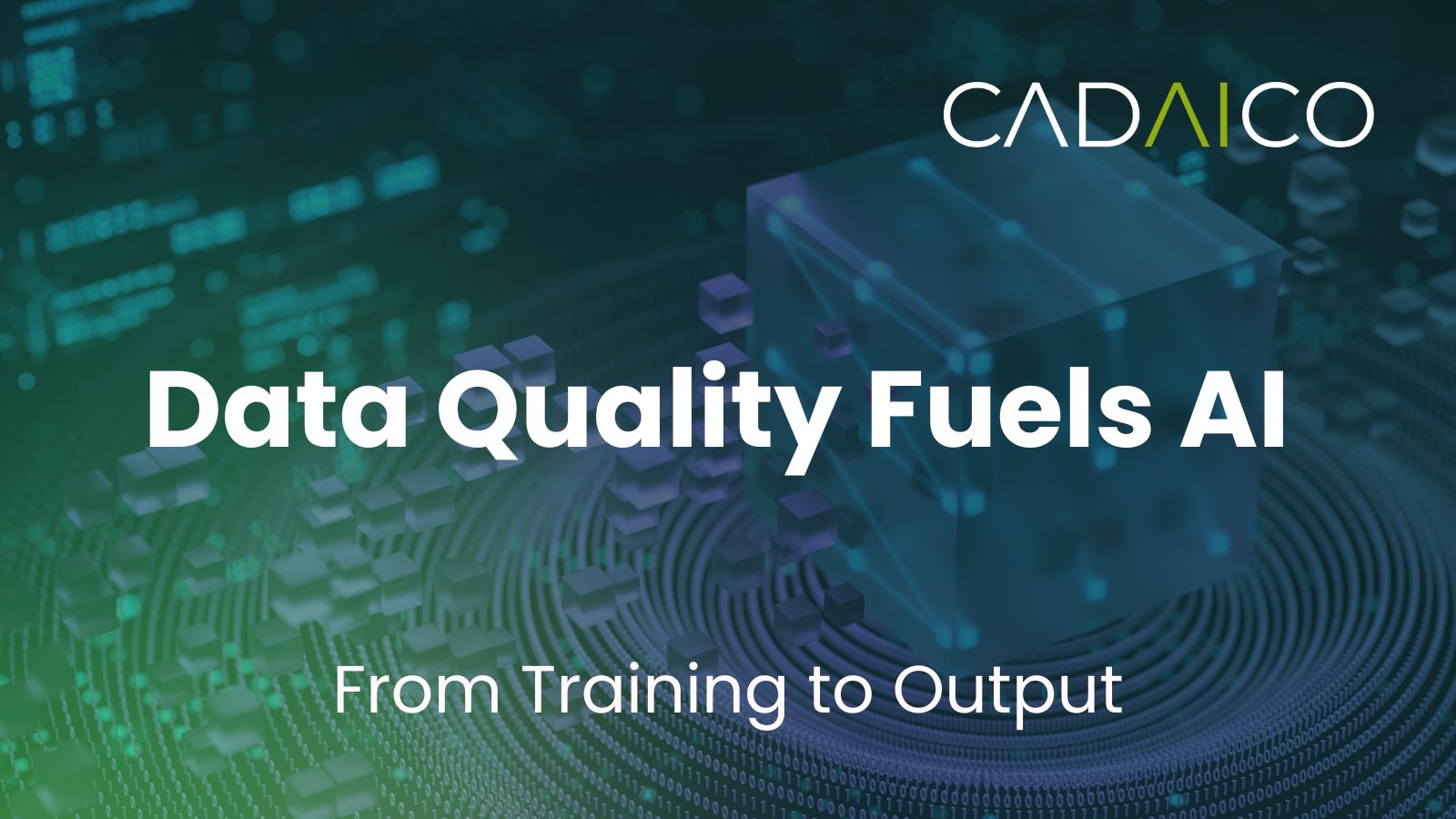
Why Data Quality is the Lifeblood of AI: The Key to Training and Output Excellence
In the world of artificial intelligence (AI), the phrase "garbage in, garbage out" couldn’t be more accurate. At the heart of every AI solution lies data—the raw material that shapes its learning, decision-making, and ultimately, its value. For businesses adopting AI, the quality of data used in training is not just a technical consideration; it’s a make-or-break factor that determines the effectiveness, relevance, and trustworthiness of the solution.
The Role of Data in AI Training
AI systems learn patterns, make predictions, and generate insights by analyzing data during their training phase. This training data is essentially the foundation upon which the entire AI model is built. If the data is incomplete, inconsistent, or biased, the AI model will inherit those flaws, leading to inaccurate or unreliable results. On the other hand, clean, comprehensive, and representative data enables the AI to deliver outputs that are precise, actionable, and aligned with real-world needs.
High-quality data ensures that AI systems can:
- Understand Context: Rich, contextual data helps AI models recognize subtle nuances and complexities in tasks, leading to smarter decision-making.
- Reduce Errors: Clean data minimizes noise, ensuring that the AI focuses on meaningful patterns rather than irrelevant anomalies.
- Enhance Predictive Accuracy: A well-trained AI model based on accurate data can predict outcomes and trends with higher confidence, providing businesses with actionable insights they can trust.
When data quality is poor, the consequences are significant. Imagine an AI assistant trained on outdated maintenance logs or incomplete design specifications. The recommendations it generates could lead to costly errors, increased downtime, or inefficiencies—undermining the very purpose of adopting AI.
The Power of Company-Specific Data
For businesses, using AI models trained on generic datasets might seem like a quick win, but it’s a limited approach. Generic data lacks the specificity needed to address unique organizational challenges. This is where the importance of company-specific data becomes clear.
When AI solutions are trained on a company’s proprietary data—be it historical production logs, maintenance records, or unique design parameters—the outputs are not only more relevant but also more impactful. Such tailored training allows the AI to:
- Adapt to Business Needs: Custom training ensures the AI understands the company’s specific workflows, tools, and industry nuances.
- Improve Operational Efficiency: Solutions built on internal data are more likely to streamline processes effectively, saving time and reducing costs.
- Deliver Competitive Advantage: Proprietary insights generated by AI models trained on unique data give companies an edge in decision-making and strategy.
Connecting Data Quality with Blockchain
Data quality and trust go hand in hand, and CADAICO leverages blockchain technology to ensure both. Blockchain can provide an immutable and transparent record of data provenance, quality, and usage, addressing one of the key challenges in AI: trust in the data foundation. By recording where the data comes from, how it’s been processed, and when it was used, CADAICO will create a verifiable trail that builds confidence in the integrity of our AI solutions.
Moreover, blockchain can ensure in the future that sensitive company data is protected while enabling secure collaboration. Companies can share high-quality datasets without losing control over proprietary information, fostering innovation without sacrificing security. This decentralized and tamper-proof approach guarantees that AI models are trained on validated, trustworthy data, resulting in more accurate and reliable outputs.
By embedding blockchain into its ecosystem, CADAICO not only enhances the quality of its AI training but also provides businesses with the transparency and security we need to adopt AI solutions confidently. It’s not just about using better data—it’s about creating a system where data is always trusted and its value fully realized.
The Data-Output Correlation
The quality of an AI model’s outputs is directly tied to the quality of its training data. This correlation becomes even more critical as AI systems evolve to handle complex tasks. For instance, an AI assistant designed to diagnose machine malfunctions will only be as effective as the diagnostic records it was trained on. If the training data is accurate and detailed, the AI will deliver precise solutions. However, if the data is riddled with gaps or errors, the AI’s recommendations could lead to misdiagnoses and operational inefficiencies.
Continuous feedback loops also play a pivotal role in maintaining output quality. By incorporating real-time user inputs and updated datasets, AI models can refine their understanding and deliver even more accurate results over time. This iterative improvement relies heavily on ensuring that the incoming data remains of high quality.
Building a Data-Driven Future
Investing in data quality is not just a technical priority—it’s a strategic imperative. Businesses that commit to maintaining clean, accurate, and relevant data unlock the full potential of their AI systems, creating solutions that are reliable, scalable, and uniquely suited to their needs. At the same time, companies must foster a culture of data stewardship, ensuring that data is not only collected responsibly but also used effectively to drive innovation.
With AI poised to revolutionize industries, the importance of data quality cannot be overstated. It’s the foundation upon which successful AI solutions are built, and the key to achieving transformative outcomes in a competitive world.